Precision Agriculture and the Costs of Inaccuracy: An Interview with Oane Visser
Interview Transcript
Precision Agriculture and the Costs of Inaccuracy: An Interview with Oane Visser
Interviewer: Aidan Smith Interviewee: Oane Visser
Text has been edited lightly for concision and readability.
Visser: With this idea of the precision trap, what I mean is that when there is an over optimistic belief in the accuracy and the reliability of those new technologies, the farmer and other actors do not observe and corroborate and check on things anymore as much as they did in the past. So then there’s the problem that if the technology is not so precise as it’s claimed to be that diseases and other problems are spotted too late in the game.
[Musical intro]
Smith: Welcome, my name is Aidan Smith and I’m a research fellow at the Center for Law, Energy, and the Environment at the University of California, Berkeley. You’re listening to the second interview of a three-part interview series where we’ll be exploring the effects of digitalization on environmental governance. I use environmental governance as a lens for understanding the actors involved in environmental decision-making. This lens also brings focus to the ways that environmental problems are framed and acted upon and whose interests are prioritized, and whose are marginalized. Digital approaches have become more and more prevalent as a means of addressing the growing concerns of environmental degradation and climate change. I had the pleasure of speaking with three social scientists who are examining these new modes of digital environmental governance. Through these conversations, key themes emerge that can help guide the public and decision-makers as they think about the role of technology in their lives and in their work. So, with that, let’s continue with the second interview.
[Musical interlude]
Smith: Welcome. I'm so excited to be joined today by Oane Visser. Oane is an Associate Professor in Agrarian Studies at the International Institute of Social Studies. His research focuses on digital technologies within agriculture and development, large- and small-scale farming and the financialization of agriculture, and alternative food networks and rural movements. Oane is an anthropologist by training, having earned his PhD from Radboud University, Nijmegen, in the Netherlands.
Oane joins us today to talk about his most recent research on precision agriculture in the Netherlands, the U.S., Canada, and the U.K. Precision agriculture goes by several other names including, “smart farming,” “agriculture 4.0,” and “digital agriculture.” The title of his published article is, “Imprecision farming? Examining the (in)accuracy and risks of digital agriculture,” which was published in the Journal of Rural Studies.
In this conversation, we’ll be speaking about how digital technologies are being deployed on farms and to what end. His work speaks to questions of digitalization and environmental governance and the challenges and risks associated with the increased prevalence of algorithms in agriculture and environmental spaces more broadly.
Welcome, Oane. Thank you for joining me.
Visser: Thank you.
Smith: I wanted to begin by having you describe, in your own words, what is your research about, and what is the scope, the focus of the research, and the primary research question that you're looking to address?
Visser: I have different sets of projects around digitalization of agriculture. My main fascination is how this whole digitalization, automation, and the rise of artificial intelligence — this whole set of technologies — how they change the world.
One big question is on the intersection of environmental change, climate change or, as people increasingly argue, climate crisis or climate destruction, and digitalization, and how those two things relate.
I'm very fascinated by this process of digitalization and the rise of the Internet of Things. How can they help us as humanity to cope with this crisis, particularly in agriculture, but that is an entry point to the environment at large.
Smith: And could you describe what precision agriculture is and how it works? What problem is it intended to solve? Advocates of precision agriculture, they have this vision of what it can do
— this digital transformation — what it does for farming. And so, I'd love for you to just describe a little bit what it is and what is the problem it’s meant to solve.
Visser: Precision agriculture under its different names is, by proponents, put forward as the way to solve the main problems of conventional industrial agriculture. It promises to go away from the very industrialized, standardized way of agriculture with monocropping, where fields are, or livestock are, treated in the same way, in a standard way. So, we have big stretches of land with corn and they all get the same fertilizer. They are sprayed with the same amount of liters or gallons of chemicals, et cetera. And then, precision farming comes in — precision agriculture — it has the promise that we move away from that model, that you can be much more accurate by being data-driven, by generating a lot of data on crops. You can give exactly the needed amount
of fertilizer, of chemicals. So, the idea is that the whole agricultural system becomes way more efficient and that also the environmental downsides become much less, because you avoid over application of fertilizer, chemicals, et cetera.
Smith: And water and energy.
Visser: Water. Energy. Yeah, so all those aspects of agriculture as a resource intensive endeavor, precision agriculture argues that, by being more precise, we can reduce that resource intensity. At least that's the claim.
Smith: And what are the technologies or devices that go into precision agriculture?
Visser: I would say there are a few core elements of precision agriculture. One has been the rise of sensors, which can be attached to combines and tractors, put in the soil, or be mounted on milk robots. So, sensors which collect data about all aspects of agriculture.
Then, secondly, you have the algorithms and the artificial intelligence, which then analyzes the data gathered by sensors and subsequently gives advice to the farmer, or even — when it's a highly automated farm — directly instructs robots to do certain applications in the field, for example.
So, these are the core elements of the Internet of Things as we see it also in, say, the manufacturing industry. But, in addition, I think a key element is that because it's outside in nature, in the fields, GPS is also a core element. So, it's also about the location of the sensor, the location of the data. That's very important for being precise.
And then subsequently this set of tools can be used in all kinds of combinations: in milk robots, which analyze everything about the cow while milking the cow, or combined with harvesting crops like grain or corn. But at the same time harvesting huge amounts of data, which immediately go into the cloud.
Smith: Thanks for laying that context, that background out there. Could you describe who the main actors or forces are that are driving this trend towards precision agriculture and where is it being deployed and implemented?
Visser: So, first about the actors. At first instance, it seems like a very complicated field or a very diverse field with lots of new startups, new names popping up every year. But if you look beyond that, like what are the companies who really acquire those startups once they start to have some impact, get some size, then basically we see three sets of actors.
First is the AgTech manufacturing industry. So, the traditional, big manufacturers of tractors and combines, like John Deere, AGCO, those big companies. Also, the companies in milk robots.
That's one set. So, they acquire various startups and are making this transition from just equipment to cloud-based tractors and combines with lots of sensors on it.
The second set of actors is also from agribusiness at large. Those are the chemical companies. Especially, you have the fertilizer companies, but most of all those providing pesticides and herbicides. So, Bayer-Monsanto and Syngenta and those kinds of companies. They even invest more money than the first group.
And then, thirdly, more recently, we see the big tech firms from outside agriculture coming in — the big names we know like IBM, Google, and Microsoft. They suddenly also obtain startups, start developing new apps for agriculture, et cetera. So, basically, huge corporate interests from different sides all jump on this.
Smith: And then geographically where is this being applied?
Visser: Basically, precision agriculture originated from the U.S. In the ‘90s it started developing, but it was still an infant industry. And so, the U.S. is still a prime actor and a prime country where it’s being applied, with Silicon Valley and the Midwest. And then we also see the E.U., especially the west. The E.U., like the Netherlands, Germany, the U.K., France, are big players. And then, aside from that, these are the core areas with Australia and Canada. Then we also see smaller hubs of digital agriculture in the Global South or emerging economies. So, we see some of the big farms in Brazil and Argentina. The same in Eastern Europe and Russia and Ukraine. And, in a very different way, we see it more targeted at smallholders via apps, et cetera. We see it also happening in Asia and Africa more recently. So, it's almost across the world, but really most far advanced in the West.
Smith: And did you say that the target or the focus is primarily smallholder farms or large scale? Or does it depend on where in the world you're looking in terms of farm size or types of farms that are being pulled into this transformation?
Visser: It depends a bit on how you evaluate it. But, if you take money as an indicator, and it’s always quite relevant where most of the money goes, in terms of investment in this industry, it's definitely the big farms, the mid-size and big farms, like in the Midwest in the U.S., some parts of California, Northern France, and the Netherlands. And the smallholders get way less investment.
Smith: I was looking recently and saw that in 2020 there was an article about how the University of California, Merced joined the University of Pennsylvania, Purdue University, and the University of Florida in forming a research center on the Internet of Things for precision agriculture. And then I read an article that in 2022 UC Davis now also has a minor in precision agriculture. They write on their website that, “Precision agriculture is the latest farming concept that optimizes fertilizer, pesticide, and water use, while minimizing the environmental concerns.” So, it seems a lot of the universities in California are picking up on this trend. I am curious, do you have any insights into the U.S. or California in terms how digital technologies are penetrating these areas?
Visser: We see that in the 1990s when it started, and in the past twenty, thirty years, the main focus has been on the much bigger farms in the Midwest, like the core staple crop areas with corn and soy and wheat. So, this has been the focus and is still the main focus.
And you also see — we can probably discuss this later — that most of the technologies are also oriented at those crops. But, at the same time, in recent years, we also see some expansion into other crops like fruits and vegetables. California comes in, the Netherlands with a lot of vegetables. And we also see that currently quite a bit of money in Silicon Valley goes to things like the development and design of robots to pick strawberries, for example, and other fruits. So, that's definitely picking up in the past few years.
Aidan: In thinking about the role of the farmer in all of this — obviously a very important actor in this whole system — I want to get your sense, again, of what is the driving impulse? What is the motivation for using digital technologies in farming? One of the narratives I've come across has a lot to do with climate change and the challenges that climate change poses to farmers. So, I want to get your reaction to this narrative. I'll lay it out for you and I would love to hear your thoughts. So, the narrative goes like this: due to climate change, there's a greater level of volatility with weather and with environmental conditions. And so, while local knowledge and expertise, held by the farmers was adequate in the past, it's now no longer really sufficient to meet the moment. In addition, population growth, market volatility and crop epidemics compound the instability wrought by climate change and will produce their own shocks. And so, digital technologies allow farmers to meet this increased volatility with more precise, accurate and reliable data. And I'm curious to hear how you respond to that narrative and these claims? Does it bring up anything for you in your own research?
Visser: I think this is definitely a narrative which is very widespread amongst proponents and policy makers. And I think it's problematic in various ways. First, it really describes the farmer — frames the farmer — as the problem for development and adaptation of agriculture. One CEO of one of the big AgTech companies said that the key bottleneck in digital agriculture is the farmer. So not a very pleasing view of the farmer. But then, if you look into the history of farming, there have been lots of moments in history where farmers have adapted to changing circumstances. Before climate change, there could be population growth, there could be droughts, other disasters, and agriculture has often adapted and farmers show a lot of ingenuity and improvisation.
Secondly, I think that what's problematic about this view is that, where farmers have a hard time adapting, this is to a large extent actually caused by earlier technologies, not by farming styles inherently. So, we see that a lot of farmers have become so indebted to finance — new, bigger machines, bigger tractors — this makes it hard for them to experiment with other things because they’re so in debt because of earlier technologies they bought, and it makes adaptation difficult. It becomes very path dependent. They're kind of determined by their stables, their barns, their tractors, with all those investments engrained in it. That's the second problem.
And the third, it poses a too optimistic view of technology in my view. So, technology is really posed as very accurate, reliable, and also kind of flexible, at the same time. And during my research, it was really intriguing for me to find that a lot of those technologies, certainly in the view of the farmers, were not as reliable as they’re claimed to be by their proponents. So there are quite a lot of inaccuracies. There is a lot of talk about “sub-inch” accuracy and then, when you look at it, that doesn't materialize in practice. So maybe there is a sensor which can sense, or the GPS can be as precise as half a centimeter or something, but then when you have implements, which have a width of 12 meters, then what can you do with that data, which is so precise? Or, subsequently, when the algorithm doesn't use that precision, there are errors introduced. So this accuracy is one problem.
In terms of the technology and the overestimation of what it can do, the problem becomes: can technology keep up with the changes in the environment, with climate change? Is it catching up? Is it keeping up with the base of climate change? That's still a big question mark.
Smith: Presumably the algorithms need to be fed some dataset to function. And so, I think in a previous conversation, you had mentioned that the normal or the baseline that these algorithms are working from is a moving target. And I'm curious if you have any other reflections on that or is there a sort of power in human ingenuity to adapt? Can you speak to the datasets that actually drive the algorithms that are so core to precision farming?
Visser: There is one problem with adapting to climate change, which — based on these new data-driven, digital models — is that to analyze or even to predict you need a historical data set, which is useful for the coming year, the present year. And I think that's a problem. So when, for example, the software by Monsanto was introduced, it mostly had just two years of data, two years of history, which even according to a lot of experts is too little. You would need at least five years to have a bit of an average, because you had so many fluctuations in agriculture year on year. And that then compares with farmers, who may be in business for thirty years, forty years, and who know all those fluctuations and keep that maybe intuitively or have scratched it down in their notebooks.
And then, with climate change it becomes even more problematic that data solutions are always the magical tool to solve this issue of adaptation, because you can wonder, even if you have five years of data, is it still helpful when climate change constantly changes the normal — moves it to new normal. Historical datasets become super quickly outdated. We see that with the weather and the IPCC reports, which are constantly running behind the reality. Climate change constantly surprises even those researchers. So, that same problem definitely is haunting precision agriculture. And during conversations I had, for example, with scientists in climate mathematics — quite a new field, for example, at Cornell University — what I find fascinating in terms of say weather prediction — just the normal weather we see on the news — is that in the past few years, technology — the models — hardly have made any progress. So, when you go to a week or ten days ahead, we hardly know if you go beyond ten days whether it's even going to rain or not. Let's not say we have any precision of the amounts of it, and it seems almost impossible to develop further. So, the same might be with climate change. The idea is that
technology gets better and better, so we’re getting closer and closer to the truth, we're getting more and more precise. But that assumes that reality is a kind of fixed state, but we see that there is an acceleration of change in the environment with climate change.
So, it's really a race between technology and climate change, and with climate change not being a linear process — but accelerating — even with those talks about technological development, which is accelerating, even that poses the question, does it go quickly enough?
Smith: I want to start shifting to a conversation around what this all looks like on the ground. We've been talking about this already a little bit, but I wanted to pull out a part from your paper where you discuss this conversation between social scientists and the engineers and the computer scientists who are developing the algorithms. You write that the critical literature on big data has historically tended to focus on human-technology interactions within “online” spaces — such as social media and the internet — and that this literature has critiqued the claims of objectivity and accuracy, which are commonly associated with big data, artificial intelligence, and algorithms. And then you note that now there's this growing interest in studying these claims in “offline” environments, such as the farm, where claims of objectivity and accuracy become probably, I would guess, even more tenuous. And so, could you explain or describe some of the challenges that “offline” spaces and environments pose to digital technologies? What are some of the factors that affect the accuracy of these tools on the farm?
Visser: I think that’s a really important topic because a lot of researchers take our online realities — and the problems we face there or the possibilities — as a starting point, because 95% or more of the literature on digitalization has focused on that in the past years. Although there are also problems of inaccuracy, the problem is much less than when we go “off screen,” so to say. When you have human behavior on the internet, it basically is sent to the algorithm in quite a pre-structured way. By just typing, we send in very clear messages and, moving with our mouse, it's all kind of translated by the computer in zeros and ones, and it’s all getting into the system in quite a structured way, unmediated by the rest of the environment.
When we go into agriculture and into the environment, but also in smart cities, then we see that a lot of other aspects of the context certainly start to intervene — to affect the quality of the data. Like in cities, you might not have the right reception everywhere because of big buildings, et cetera, going into tunnels, if you would track human behavior, mobility behavior. If you go into nature it even gets more difficult because plants act in sometimes unpredictable way.
Animals start also to react, sometimes, on sensors. They destroy them. Or, I heard of stories of farmers, who say, when I put a camera on a cow, sometimes they start behaving differently. So, it's not unaffected, the data you gather. And you see the weather, you see that sensors very quickly get destroyed or they lose their accuracy because of storms, wind, et cetera.
And to give an example of research we’re doing at our institute; a colleague did environmental data collection and research in the Amazon to monitor forests. They used drones and thought that was a great idea to monitor pollution, et cetera. But these drones, they had a very hard task even flying in the Amazon with all the tropical heat, et cetera. So, the batteries very quickly
degraded and the drones even had difficulty getting back to the loading station, let alone gather useful, accurate, actionable data. And we see the same in agriculture, but also in the environment widely. There have been experiments with mounting sensors on whales. And then those sensors themselves become the problem because, by being put on the whales, they cause infections. So, either the project fails or the animals start to become ill. So, there are a lot of problems, which we have to take into account when studying digitalization out there in the wild, so to say.
Smith: And these tools tend to treat the farm as a laboratory, and maybe that analog, comparison, or metaphor is not appropriate in this instance.
I was going to ask you a question about the possibilities and limitations of using digital technologies. Because every time we think up a solution for a problem that often is very tightly linked to how we frame the problem.
And so, I was curious to hear how you see this approach. What does it make visible and what does it obscure? Does it just distract from root causes in some instances? Where does it draw our attention to? And, in terms of precarity for farmers, what are some of the other root causes of hardship or struggle?
Visser: The idea of the “quick fix” with technology makes invisible the big power dimensions in agriculture and the increasingly precarious position of the farmer in this whole system. And then, by giving them new tools, there is this idea that the farmer is empowered and is on top of things. But, when we take a closer look, they often get increasingly indebted.
And even when it's technology, which is supposedly introduced for environmental purposes, like in livestock farming you have low emission floors in cow barns with valves which open and close. They would separate the urine from the rest of the manure and then drastically reduce the nitrogen emissions, for example. So, farmers put in a lot of money to install those things.
They were basically tested in the kind of lab situations you referred to earlier — like ideal situations — which were relatively clean or well maintained, et cetera. And you see in reality that by no means the environmental benefits are reached in practice, because there is the weather. There is dirt. So those installations very quickly start to malfunction or farmers don't have the time to do the necessary repairs. So, the proclaimed benefits and accuracy drop. They don't get the results they’re promised and often the farmer is blamed for that. For example, with milk robots, you see that they are quite accurate for say 98% of the farmers. It's quite a high number. There are some problems in the beginning for cows to get adapted, but then for most farmers it works. They're always, in each barn, some cows which cannot deal with the robots and then they just have to be brought to the slaughter. So, they don't fit. These cows are not uniform enough to fit the robot. But then there are also cases which can become quite more dramatic.
The whole herd doesn't want to go into the milk robot. And then there are farmers where it worked in the beginning, and then suddenly after some months the cows don't want to get into the milk robot anymore. So then very quickly diseases start. Infections start. So, the farmer really gets in trouble.
And then there are several cases, for example, in the Netherlands — but I guess in other locations just as well — the problem is the farmer wants compensation or wants the manufacturer of the robots to address this issue, repair it. It might be that there is electricity leaking or there are other problems inside the robot. But then the AgTech manufacturer of this technology says like, um, no, it's probably your problem. It's the cow’s problem or bad management of the farmer. And the contracts the farmer has to sign when he buys this equipment are almost a centimeter thick. So, basically, the farmer has to prove that all other aspects — like the weather, the animals, the farming style, the buildings — cannot cause this problem and that, by elimination, it could not be anything else than the robot. That burden of proof is impossible with so many factors. So, the AgTech manufacturers get away from it and the farmers get in huge trouble sometimes. I know of one case, a farmer, they were at the fringe of bankruptcy and his mother was afraid he would hang himself. So, this is an illustration of how inaccuracy, when it combines with very unequal power relations, can be really dramatic for the farmers.
Smith:
Thanks for sharing that. And I would love to, maybe a little bit later, dig more into those power relationships. I did want to ask you — because this is an interesting concept that comes up in your work — is that you look at how the farmer actually plays a very important role in these tools. They play a very important role in calibration, corroboration, and interpreting what the tools are telling them. And so, the user of these tools is so important in actually how effective they truly are. I was wondering if you could speak to the role of the farmer in employing this technology. What are some of the instances or examples that you can draw on there?
Visser: Here again there is quite a contrast between the rhetoric of those sensors — the technology being super precise — and the reality. I found that farmers have to make them precise to really make them work. So, there is quite a lot of labor from the side of the farmer needed.
One thing is that, if you have a sensor that measures the yield — the yield of say a combine, the yield per square meter — then you can get, at the end of the harvest, an overview. Like, what are the high yielding parts of the field? What are the lower yielding parts of the field?
And that helps the next year. And you can adapt your application of fertilizer, et cetera. So in theory, that's great data. But those sensors have to be calibrated. That has to be done by the farmer. So that requires some work and experience. But then also, once the farmer is driving, he has to keep an eye on, do I get data that seems logical or not? One farmer mentioned, for example, that suddenly when he started driving a bit more quickly, suddenly the yield per square meter went up. He slowed down and it went down. That shouldn't be like that. There seems to be a bug in the system. And you also see that, for example, with harvesting potatoes or sugar beets, et cetera, it could be that there's a lot of tare, a lot of mud, et cetera. And then the system thinks — the sensor thinks — that it's just a lot of potatoes.
And in all those instances, the farmers have to keep an eye on it and think, is this logical or not? Does this make sense? So, it's not just driving, putting on the switch and it's all done. It's a lot of corroboration in the field, observation, critical thinking based on experience.
Smith: I want to shift to some of the more conceptual parts of your paper and of your work. You argue that an over-reliance on big data and algorithms — and you've done a fantastic job of explaining all the different contingencies and factors that we should be thinking about when using these technologies, and being cautious around the conclusions we draw from these technologies — and you write that an overreliance on big data and algorithms can lead to what you call a “precision trap.” And so, I would love for you to explain what you mean by a “precision trap,” and why they present a potentially growing problem.
Visser: With this idea of the precision trap, what I mean is that when there is an over optimistic belief in the accuracy and the reliability of those new technologies, then there is a tendency to lay back, as a farmer or a farm consultant, like, okay, this technology is so great. It does the job and I don't have to go in the field once a week as I did before. I don't have to check on the cows like I did. So, there is a big risk that by having such a strong belief in the accuracy of this technology, the farmer and other actors do not observe and corroborate and check on things anymore as much as they did in the past. Like when the farmer was milking cows himself or herself, then daily you see every cow and you spot things — diseases, strange behavior, et cetera. So, the promise of this milk robot is that you don't have to go in the barn every morning, every evening anymore. So, then there’s the danger that a farmer hardly goes in the barn anymore. He or she relies totally on the data and the printouts on the cows. So, then there’s the problem that if the technology is not so precise as it’s claimed to be diseases and other problems are spotted too late in the game. And there might be a lot of negative knock-on effects in terms of diseases, in terms of harvests that are lost. So, I think that's a big problem and especially this problem when the technology also claims to predict things. So, there are new technologies, new sensors, which are sometimes based on satellites, that create images of the crops during the time they’re growing. And then it claims to detect diseases before they can be seen by the farmer. So, then he or she can start spraying much earlier and arguably prevent a much bigger outbreak. But when the algorithm is wrong, it could be that you're just spraying additional times. And when there is an error, there might not be a danger of a disease at all. So, you're just polluting the environment, making additional costs. And it's of course much worse when you don't see a problem, a disease. You think, okay, I don't have to go in the field anymore. It's just spotted by the algorithm, by the sensors. So, there is a big risk of failures with different side effects.
Smith: In your paper, you write that your work is unique in that a lot of previous work in this area takes as a given that these tools are precise, and — correct me if I'm wrong — but your work challenges that assumption that these tools are actually accurate, right? So, this is how it deviates or is unique in the literature, because other scholars take that as a given and then draw conclusions about what that means for society.
Visser: I think one problem of the technology is (which other authors have stressed, like when there’s a lot of precise information about a farm by other actors — the big, broader surveillance trend, which is also definitely happening) the precision, the amount of data gathered, et cetera.
That is a mounting problem, but that's still often something still for the future, because a lot of those technologies are not as precise as they are claimed to be. So, the bigger problem, in my view, is not that it's this all-knowing technology, which is also scary, but the problem is that we think it's an all-knowing technology and it isn't. So, it camouflages a lot of potential landmines, on the way, because we think it's so super sophisticated.
Smith: And, in your paper, you write that it's not so much that we should have the expectation that it’s a perfect tool. It's more the humility or this knowledge that the tool has some utility, but it's important to know the limitations of the tool. Is that accurate?
Visser: Yeah, absolutely. So, I'm not a Luddite in the sense that I'm against those technologies. I definitely see the value in various ways, but I think it will become quite dangerous if we have this excessive belief in things it can do when it doesn't. We saw that also in other terrains, like in the airplane industry with the Boeings, where a new system was introduced and they thought it's so accurate, so we hardly have to train the pilots. They don't have to know what the new system is about or they get a very quick onscreen instruction. And that appeared to be very dangerous with crashes or near crashes. So, again, this overestimation of technology…that's a big risk.
Smith: The other big conceptual piece I found in your work was a slightly different term. You talk about the “precision divide,” and I think that that speaks a lot to the biases that are inherent within the technologies themselves. And so, I would love if you could describe what you mean by “precision divide”? How does it arise and what might be some of the repercussions of this divide?
Visser: I think it’s important, first of all, to distinguish this from the digital divide. So the digital divide is often like, okay, farmers don't have the knowledge to operate a computer or they don't have the money to buy new technology. So, you get a big divide between farmers who have the money and knowledge to jump on the new technologies and others who don't.
What we mean with the precision divide is that, even if the farmer has the money and the knowledge, et cetera, there may be divides which are more inherent in the technologies and exclude people. And that is more on the level of the algorithms. And that talks back a bit to this idea that for effective algorithms, for effective devices, we need data, we need a set of data, a historical set of data. And then we see that, as we mentioned earlier on, most of the technologies have focused on the Midwest, on the core crops, the staple crops. So, they have been trained on those crops. And farmers who then try to use these technologies in, say, California, for other crops, for vegetables, or in the Netherlands, then certainly they run into problems, not because they have a lower level of knowledge or because they have less money. No, because this algorithm has not been trained on those circumstances. So, by definition, it's much less precise. So, there was a new farmer, for example, who stated, yeah, those
algorithms, they are a disaster in this cultivation. So, he got very disappointed. And you see that also in flowers and various other crops. So, we see that those algorithms tend to concentrate on the most widespread crops because that's the most profitable market for them. And so, it then creates a bigger and bigger divide between farmers who have relatively precise technologies, like in wheat and in corn, and those who are in other crops or in different environments using the same technologies, and they get garbage out of their algorithms, to overstate it a little bit. It becomes quite problematic.
Smith: What is this self-reinforcing nature? Why does it get worse as these algorithms learn from more and more data?
Visser: So, there’s a tendency of self-reinforcing trends, because they were first introduced in those few crops. Then most data are gathered there and then it gets even more precise and more farmers become drawn to those crops. So, more and more years of data are piling up. Whereas for other crops, they are getting further and further behind, with the farmers who are cultivating them. It's a bit like if you have a search engine like Google and a new startup tries to come in with a search engine. Google has been trained for all those years with billions of users and it's crowding out all those other algorithms.
Smith: And in terms of the precision divide, do you find that the algorithms also privilege certain types of farming practices, like permaculture or integrated crop farming. Is that also a point where you see difference and divergence?
Visser: Yeah, absolutely. So, this precision divide is not only about crops, but also about the farming system. So, when you're doing just corn and alternating with another crop, that's something which can quite easily be captured by the algorithm. But if you're doing a lot of crop rotations, it's more difficult to build up this dataset for each crop. So that's already a problem.
And, secondly, I also did interviews with farmers in regenerative agriculture who try to have lots of different crops in rows on their field. Then the problem is that the data sometimes got influenced by crops nearby. It's difficult to collect data for each crop, because it needs almost a different machine and a different software package for all those different crops. Then you have different machines in addition. So, the system of digital agriculture as it is now is very much focused on and reinforces this idea of monocropping. And when you want to do much more experimental — other styles of farming — it's very difficult to do so. Not impossible, but very difficult.
Smith: In thinking about digital technologies, another idea that comes up in lots of different areas is this idea of real-time, algorithmically-driven, environmental decision-making. And, for many, this has a large appeal, right? But then others argue that this kind of automated
decision-making, in its speed, can foreclose political debates. It can also have other unforeseen ramifications. And so, this idea of real-time, through algorithms, what do you make of it and what do you think is important to consider or highlight when we think about the speed at which these decisions are made?
Visser: Yeah, this is a very appealing part, at first instance, obviously. And it also has a lot of benefits, but also, at the same time, a lot of risk. Because, paraphrasing one of the farmer sources, he said, like, okay, we have this new algorithm, this new technology we use in the field, which generates a lot of data — just real-time, in split seconds. It sends lot of useful data, but sends also a lot of errors at the same time with lightning speed. So, there is also a risk that because it's so real-time, you have hardly any time to check it as a farmer. And also, the errors very quickly ascend and go undetected. So that's also a big problem. And this example was just one device — measuring, for example, the yields. But once digital agriculture evolves further and you have different sets of real-time operating technologies on the farm and they start even interacting with each other, which is the ideal with visions of the Internet of Things, then those errors could interact with each other and result in a cascading of errors with quite dramatic fallouts and failures for the farm. So, possibilities, but also risks there.
Smith: And this gets back to what you were talking about in terms of the precision trap. By having it be real-time, this circumvents the corroboration that a farmer might engage in. So, this idea of real-time is very much linked with your thinking on the precision trap.
You did talk with us a little bit before, but I think it's so critical to the discussion, that digitalization so often reshapes power dynamics, relationships, and dependencies between different actors. And so, I'm curious, what do you see forming? Are there new actors brought into this space? I know you touched on some before, but I would love to hear a little bit more about these power dynamics — questions of access to data, ownership of data. Where do you see these tensions crop up? No pun intended.
Visser: There is definitely the management of the farm, which was traditionally firmly in the hands of the farmer, and over the years has devolved. But, we see with digitalization, that increasingly there is a kind of off-farm choreography, as one researcher called it. That more and more actors from Big Tech, from the AgTech manufacturers, they start to look at the data collected by the farmer, start to have an influence on it. If you look at the example of combines, John Deere combines, increasingly they're digitally locked. So, a farmer normally had the agency to repair his own combines and tractors in the field, et cetera. Now that's impossible.
And you first need a code to unlock the system. Otherwise you cannot do anything. You can maybe still change a tire, but that's about it. So, it's a huge dependency. Imagine you’re out there on the prairies and the help desk is closed and your harvest has to get in because the rain is coming. Or a farmer who had a constant beep in his combine and he could not solve it because it was all digitally locked. And he was just sitting there with this annoying noise.
So that's definitely outsiders getting more control over the farmer. And that also gets a transnational dimension. You see that in, for example, Africa, new platforms and new apps are being introduced, but it's often private equity, Western investors who are behind this. So, you see that increasingly value generated on the farm is extracted by outsiders and even on a global level away from, say, developing countries to financial centers, which it's also quite a troubling
tendency. This is very different from the view of those farmers leapfrogging and making progress and development because of those tools.
Smith: I think one of the common narratives of development practice and theory is that information communication technologies enable greater connectivity, right? And that connectivity as a concept is very much tied with this idea of development. But, so often, increased connectivity can also produce relationships where there are opportunities for extraction and exploitation of value, like you said.
So, as we wrap up, I did want to ask you, through your research, have you arrived at any conclusions or otherwise about how digital technologies might be better designed and deployed in our lives? And have you come across any exciting developments in the field? Is there a movement towards a more equitable, just, or sensible use of these technologies?
Visser: Luckily, there is. I mean, it's still a bit under the radar. But we see movements where precision agriculture is really designed and modified by farmers themselves, in alliance with engineers or software designers, or by some farmers who came in from a job, say, in an ICT firm, started farming, and take those skills with them. So, what we see in the U.S., especially in the Northeast and New England, but also some spots in the Midwest and in France, are movements of farmers who’ve built really exciting new coalitions with designers and ICT specialists. And based on the idea of open source, agricultural technologies, they start to innovate bottom-up and build tools that are much more adapted to farming styles, which are ignored by the mainstream corporate technologies. So, we see farmers, say in New England, who have pasture-based, livestock operations or agro-ecological operations, who build tools in hackathons, with groups of farmers and ICT specialists on the farm and design new tools, both in software and hardware, and then put that online within those movements — movements like Farm Hack and GOAT, which is the Gathering for Open Agriculture Technologies in France. You have L’atelier Paysan — the Workshop of the Peasant — so to say, of the farmer. And these are movements that are gaining more and more members and building up a repository of open source technologies, which farmers can use and modify. So, this is an encouraging technological development. And it's encouraging both in terms of more equity for the farmer, and in terms of allowing technologies to be more adapted to new farming styles, more sustainable farming styles.
Smith: Do you see your work serving that audience? Or, who are you hoping to influence with this research?
Visser: I ideally hope to reach various audiences. The interesting thing is that this paper on precision agriculture — more than any other paper I've written — has been read by people in the technological sciences, which is encouraging. Also, for example, some people in
agro-robotics have become fascinated by this. It would be great if designers became more aware of the risks. But I would also like to reach some policy-makers and the farmers’ movements themselves, which are now emerging. I think some of the farmers are not aware of how quickly some of these developments are happening, i.e. enclosing the datasets of farmers
and the very quick expansion of some of those Big Tech firms in this area. Quite a number of farmers are not aware of that yet. So, one of my ambitions is to try to reach that audience — to make them aware of both the threats and risks, but also some of the possibilities, in terms of alternative models of building technologies for agriculture.
Smith: It's great to hear that you have a focus on policy-makers as well, because I could see if you're developing a policy or a program it's really important to be aware of the limitations and the opportunities of these technologies. So, I see your work being very helpful and really providing a lot of key insights for that audience as well.
Are there any other projects that you are working on that you'd like to mention? Or what do you see as the future of this work? Or, are you focusing on other things next?
Visser: My previous work has been on open, arable farming. My recent project is more in horticulture — greenhouse-based horticulture — and there is also a lot of data there. And in terms of climate adaptation, there is also this tendency, okay, maybe we just give upon adapting. We just build greenhouses and indoor farms and shut agriculture off from the environment. So, we create our own internal environment. And whether that is in the U.S., close to New York, or whether it's in Dubai, at forty degrees Celsius, we will just build our indoor farms. So, there is this idea of total controllability of agriculture, which I think is problematic. This is one area I'm trying to look into — now first in the Netherlands, in the greenhouse clusters.
And the second, recent project, which is in Africa and Ghana, is looking at how more app-based digitalization, targeted at smallholders, is unfolding.
Smith: I could see, as we mentioned, there's this drive within precision agriculture to basically treat the farm like a lab. And so, let's put it into a greenhouse and create that laboratory environment so that our tools can work. It'll be very interesting to see if many of the same conclusions in your work on precision agriculture still apply or how that story unfolds.
Well, thank you so much. You've been incredibly generous with your time. And I want to thank you for discussing your research with us. It's a fascinating look at how digital technologies are being deployed in environmental spaces and it raises a lot of interesting questions around environmental governance and the role of digital tools in environmental governance. So, there's a lot to learn from your work. And, again, I want to thank you and I, for one, look forward to your future research.
Visser:
Thank you. It was an honor and a pleasure to join this conversation.
[Musical outro]
Creators and Guests
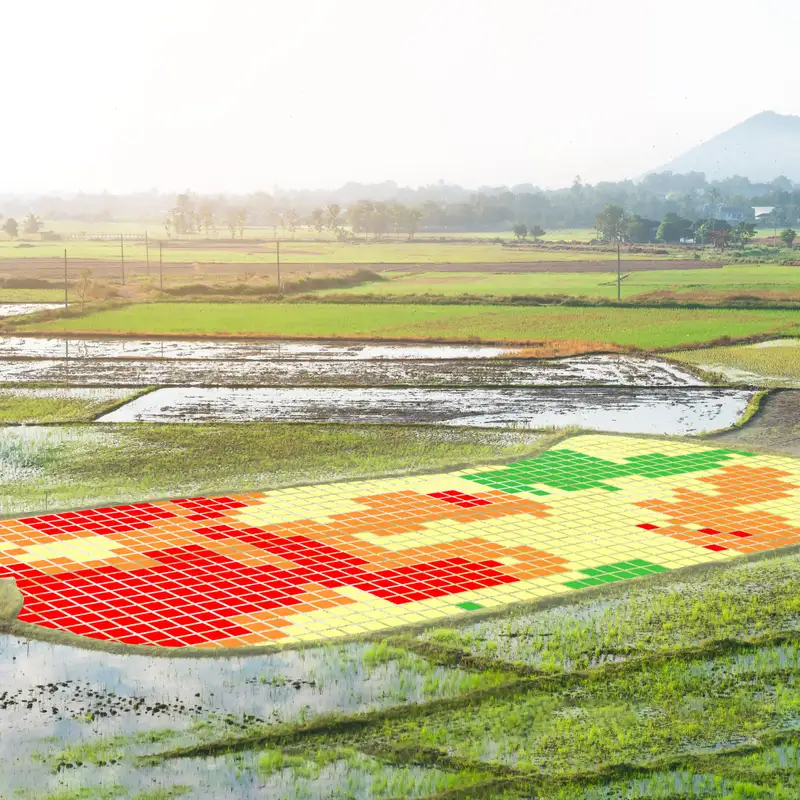